Contest | NTCIR16-FinNum3
Projects ·This is a page about “IMNTPU at the NTCIR-16 FinNum-3 Task: Data Augmentation for Financial Numclaim Classification”.
This paper provides a detailed description of IMNTPU team at the NTCIR-16 FinNum-3 shared task in formal financial documents. We proposed the use of the XLM-RoBERTa-based model with two different approaches on data augmentation to perform the binary classification task in FinNum-3. The first run (i.e., IMNTPU-1) is our baseline through the fine-tuning of the XLM-RoBERTa without data augmentation. However, we assume that presenting different data augmentations may improve the task performance because of the imbalance in the dataset. Accordingly, we presented double redaction and translation method on data augmentation in the second (IMNTPU-2) and third (IMNTPU- 3) runs, respectively. The best macro-F1 scores obtained by our team in the Chinese and English datasets are 93.18% and 89.86%, respectively. The major contribution in this study provide a new understanding toward data augmentation approach for the imbalanced dataset, which may help reduce the imbalanced situation in the Chinese and English datasets.
Keywords: Data Augmentation, Double Redaction, Binary Classification, XLMRoBERTa, Financial Claim
Proposed Methods:
- XLM-RoBERTa Based Model
- Double Redaction
- Translation
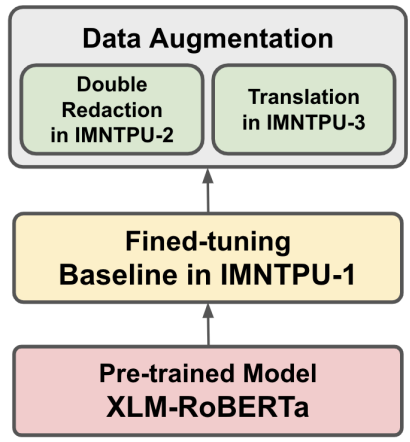