CAMLKG
Projects ·This is a page about “CAMLKG: Knowledge Graph of Cryptocurrency Anti-Money Laundering with Few-Shot Learning Model from Judicial Data in FinTech”.
With the rapid growth of FinTech and blockchain technology, the volume of cryptocurrency transactions has been increasing. Consequently, incidents of cryptocurrency money laundering have increased as well. Although legal knowledge graphs have emerged, there is little research focused on the topic of cryptocurrency anti-money laundering to date. Moreover, the emergence of large-scale language models (LLMs) has underscored the importance of understanding the differences between LLMs and other language models. Our study aims to propose the CAMLKG, a cryptocurrency anti-money laundering knowledge graph, to assist legislators and law enforcement in clarifying methods and patterns related to emerging financial technologies and cryptocurrency crimes. Additionally, we compare the performance of the large-scale language model CAMLKG+LLM with the proposed model.
This paper presents two studies: Study 1 constructs the proposed KGC model, which adopts two modules—a neighbor encoder and a matching processor—to carry out the KGC task with a few-shot learning method for predicting attributes and relations between entities from judicial data. Study 2 compares the performance of the KGC task between the CAMLKG model and the CAMLKG+LLM model. The major findings of this study, based on the experimental results, are that the proposed KGC model outperforms the CAMLKG model on the smallest size of the CAML dataset in Study 1, and the CAMLKG+LLM model performs better than the proposed KGC model on three sizes of the CAML dataset in Study 2. This study's research contribution is that it can assist in identifying potential relationships between entities from CAML-related judgments, thereby facilitating the establishment of a larger CAML database. Furthermore, it is demonstrated that LLMs outperform the proposed CAMLKG model in the KGC task, owing to the model features of in-context learning, instruction following, step-by-step reasoning, and the reinforcement learning from human feedback (RLHF) mechanism.
For the managerial implications of this study, the construction of the CAMLKG can aid law enforcement officers in determining whether new cases involve similar criminal patterns to past convictions, assisting them in making judgments.
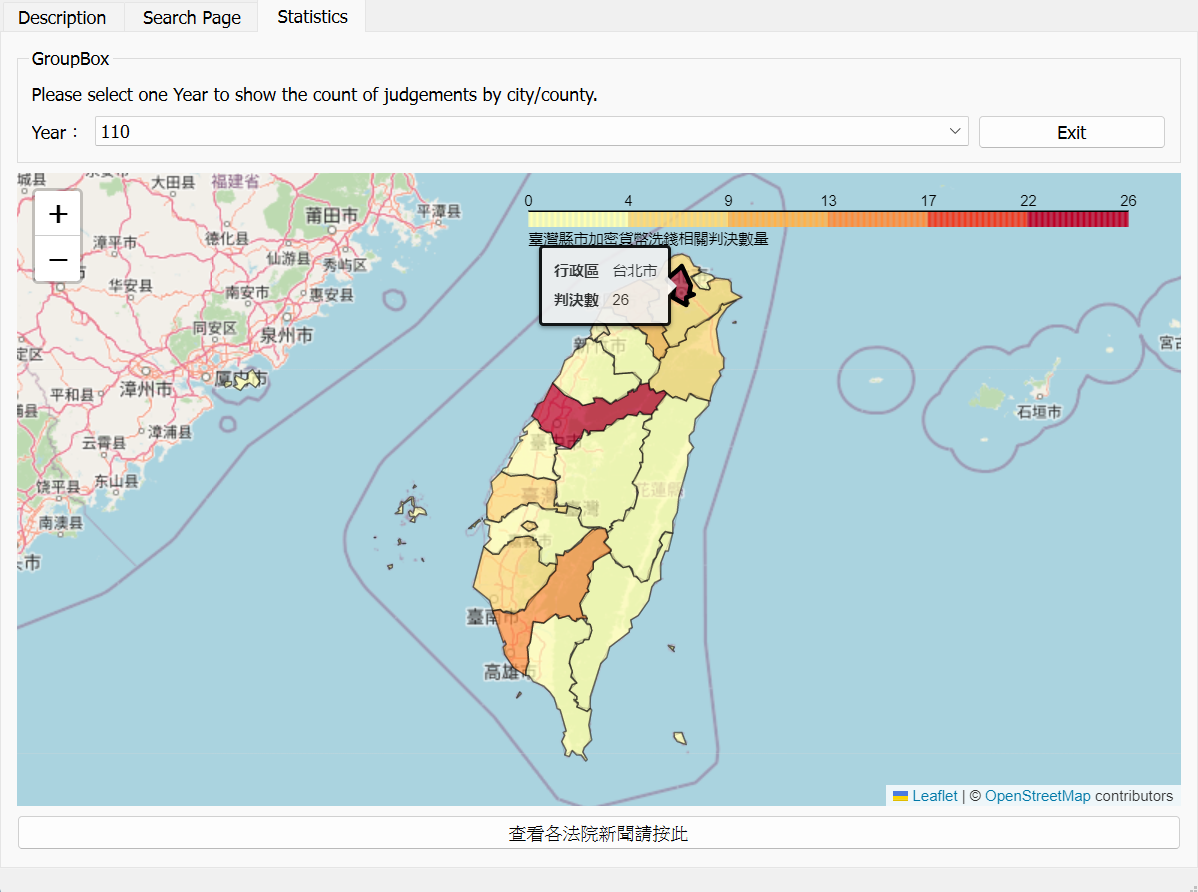